ALY 6000_Project_2
.pdf
keyboard_arrow_up
School
Northeastern University *
*We aren’t endorsed by this school
Course
6000
Subject
Statistics
Date
Apr 3, 2024
Type
Pages
18
Uploaded by MagistrateUniverseHorse40 on coursehero.com
Project 2 –
Exploratory Data Analysis (EDA) of Two Data Sets ALY 6000 Project Instructions In this two-part project, you will explore core functions within the set of libraries known as the tidyverse. Note: Utilize the file
project2_tests.R
with the code below to run a series of tests (not comprehensive) on your code. Any failed test signals that something is wrong with the results or that you have not utilized the specified variable names. p_load
(testthat)
#testthat::test_file("project2_tests.R") Setting Up Your Project Complete the following steps to create and organize your initial R project. 1.
Create a new R Project called Lastname_Project2
. 2.
Create a new R Script and save it into the R folder of your project as Lastname_Project2.R
. 3.
Download the data set 2015.csv
from Canvas and save it into the project folder. 4.
Download the data set baseball.csv
from Canvas and save it into the project folder. 5.
Download cheat sheets for the tidyr and dplyr packages for quick reference. You can access them from the help menu in RStudio. 6.
Include the following boilerplate code at the top of your file to clear the environment each time you run your complete script. cat
(
"
\014
"
) # clears console
rm
(
list = ls
()) # clears global environment
try
(
dev.off
(
dev.list
()[
"RStudioGD"
]), silent = TRUE
) # clears plots
try
(
p_unload
(
p_loaded
(), character.only = TRUE
), silent = TRUE
) # clears packages
options
(
scipen = 100
) # disables scientific notion for entire R session
7.
Include the following code at the top of your script (but below the boilerplate code) to load the pacman loader library. Then load the entire tidyverse.
library
(pacman)
p_load
(tidyverse)
Assignment Part 1 Data can measure many things. Countries, for example, can be assessed against a variety of metrics. In addition to the gross domestic product (GDP) of a given country, researchers consider other data points in assessing the quality of life across the globe. To understand how data can be wrangled to measure freedom, trust, and other measures of human life, complete the following steps. The assignment displays the expected outcome after each step. 1.
Read the data set 2015.csv
and store it in a variable called data_2015
. You can test that you loaded it correctly with the code utilizing the head function below. head
(data_2015)
# A tibble: 6 × 12
Country Region Happi…¹ Happi…² Stand…³ Econo…⁴ Family Healt…⁵ Freedom Trust…⁶
<chr> <chr> <dbl> <dbl> <dbl> <dbl> <dbl> <dbl> <dbl> <dbl>
1 Switzer… Weste… 1 7.59 0.0341 1.40 1.35 0.941 0.666 0.420
2 Iceland Weste… 2 7.56 0.0488 1.30 1.40 0.948 0.629 0.141
3 Denmark Weste… 3 7.53 0.0333 1.33 1.36 0.875 0.649 0.484
4 Norway Weste… 4 7.52 0.0388 1.46 1.33 0.885 0.670 0.365
5 Canada North… 5 7.43 0.0355 1.33 1.32 0.906 0.633 0.330
6 Finland Weste… 6 7.41 0.0314 1.29 1.32 0.889
0.642 0.414
# … with 2 more variables: Generosity <dbl>, `Dystopia Residual` <dbl>, and
# abbreviated variable names ¹`Happiness Rank`, ²`Happiness Score`,
# ³
`Standard Error`, ⁴
`Economy (GDP per Capita)`,
# ⁵`Health (Life Expectancy)`, ⁶
`Trust (Government Corruption)`
2.
Use the function names
to produce the column names for your data set. names
(data_2015)
[1] "Country" "Region" [3] "Happiness Rank" "Happiness Score" [5] "Standard Error" "Economy (GDP per Capita)" [7] "Family" "Health (Life Expectancy)"
[9] "Freedom" "Trust (Government Corruption)"
[11] "Generosity" "Dystopia Residual" 3.
Use the view
function to view the data set in a separate tab. 4.
Use the glimpse
function to view your data set in another configuration. glimpse
(data_2015)
5.
Use p_load
to install the janitor
package. Janitor has a function called clean_names
that can be given a data frame to make the names more R friendly. Be sure to store the resulting converted data frame in a variable. p_load
(janitor)
data_2015 <- clean_names
(data_2015)
data_2015
6.
Select from the data set the country
, region
, happiness_score
, and freedom columns
. Store this new table as happy_df
. # A tibble: 158 × 4
country region happiness_score freedom
<chr> <chr> <dbl> <dbl>
1 Switzerland Western Europe 7.59 0.666
2 Iceland Western Europe 7.56 0.629
3 Denmark Western Europe 7.53 0.649
4 Norway Western Europe 7.52 0.670
5 Canada North America 7.43 0.633
6 Finland Western Europe 7.41 0.642
7 Netherlands Western Europe 7.38 0.616
8 Sweden Western Europe 7.36 0.660
9 New Zealand Australia and New Zealand 7.29 0.639
10 Australia Australia and New Zealand 7.28 0.651
# … with 148 more rows
7.
Slice the first 10 rows from happy_df
and store it as top_ten_df
. # A tibble: 10 × 4
country region happiness_score freedom
<chr> <chr> <dbl> <dbl>
1 Switzerland Western Europe 7.59 0.666
2 Iceland Western Europe 7.56 0.629
3 Denmark Western Europe 7.53 0.649
4 Norway Western Europe 7.52 0.670
5 Canada North America 7.43 0.633
6 Finland Western Europe 7.41 0.642
7 Netherlands Western Europe 7.38 0.616
8 Sweden Western Europe 7.36 0.660
9 New Zealand Australia and New Zealand 7.29 0.639
10 Australia Australia and New Zealand 7.28 0.651
8.
From happy_df
filter the table for freedom values under 0.20. Store this new table as no_freedom_df.
# A tibble: 12 × 4
country region happiness_sc…¹ freedom
<chr> <chr> <dbl> <dbl>
1 Pakistan Southern Asia 5.19 0.121 2 Montenegro Central and Eastern Europe 5.19 0.183 3 Bosnia and Herzegovina Central and Eastern Europe 4.95 0.0924
4 Greece Western Europe 4.86 0.0770
5 Iraq Middle East and Northern Africa 4.68 0 6 Sudan Sub-Saharan Africa 4.55 0.101 7 Armenia Central and Eastern Europe 4.35 0.198 8 Egypt Middle East and Northern Africa 4.19 0.173 9 Angola Sub-Saharan Africa 4.03 0.104 10 Madagascar Sub-Saharan Africa 3.68 0.192 11 Syria Middle East and Northern Africa 3.01 0.157 12 Burundi Sub-Saharan Africa 2.90 0.118 # … with abbreviated variable name ¹
happiness_score
9.
Arrange the values in happy_df
in descending order by their freedom values. Store this new table as best_freedom_df
. # A tibble: 158 × 4
country region happiness_score freedom
<chr> <chr> <dbl> <dbl>
1 Norway Western Europe 7.52 0.670
2 Switzerland Western Europe 7.59 0.666
3 Cambodia Southeastern Asia 3.82 0.662
4 Sweden Western Europe 7.36 0.660
5 Uzbekistan Central and Eastern Europe 6.00 0.658
6 Australia Australia and New Zealand 7.28 0.651
7 Denmark Western Europe 7.53 0.649
8 Finland Western Europe 7.41 0.642
9 United Arab Emirates Middle East and Northern Africa 6.90 0.642
10 Qatar Middle East and Northern Africa 6.61 0.640
# … with 148 more rows
10.
Create a new column with mutate
in data_2015
called gff_stat.
For each row, the gff_stat
is the sum of the family, freedom, and generosity values. Store the resulting table right in the data_2015
variable. # A tibble: 158 × 13
country region happi…¹ happi…² stand…³ econo…⁴ family healt…⁵ freedom trust…⁶
<chr> <chr> <dbl> <dbl> <dbl> <dbl> <dbl> <dbl> <dbl> <dbl>
1 Switze… Weste… 1 7.59 0.0341 1.40 1.35 0.941 0.666 0.420
2 Iceland We
ste… 2 7.56 0.0488 1.30 1.40 0.948 0.629 0.141
3 Denmark Weste… 3 7.53 0.0333 1.33 1.36 0.875 0.649 0.484
4 Norway Weste… 4 7.52 0.0388 1.46 1.33 0.885 0.670 0.365
5 Canada North… 5 7.43 0.0355 1.33 1.32 0.906 0.633 0.330
6 Finland Weste… 6 7.41 0.0314 1.29 1.32 0.889 0.642 0.414
7 Nether… Weste… 7 7.38 0.0280 1.33 1.28 0.893 0.616 0.318
8 Sweden Weste… 8 7.36 0.03
16 1.33 1.29 0.911 0.660 0.438
9 New Ze… Austr… 9 7.29 0.0337 1.25 1.32 0.908 0.639 0.429
10 Austra… Austr… 10 7.28 0.0408 1.33 1.31 0.932 0.651 0.356
# … with 148 more rows, 3 more variables: generosity
<dbl>,
# dystopia_residual <dbl>, gff_stat <dbl>, and abbreviated variable names
# ¹happiness_rank, ²happiness_score, ³standard_error,
# ⁴economy_gdp_per_capita, ⁵
health_life_expectancy,
# ⁶
trust_government_corruption
11.
Summarize the happy_df
data set. Your summary should contain the mean
happiness_score in a column called mean_happiness,
the max
happiness_score in a column called max_happiness
, the mean
freedom in a column called mean_freedom
, and the max
freedom in a column called max_freedom.
Store the resulting table as happy_summary
. # A tibble: 1 × 4
mean_happiness max_happiness mean_freedom max_freedom
<dbl> <dbl> <dbl> <dbl>
1 5.38 7.59 0.429 0.670
12.
Group the happy_df
data set by region. Run a summary that provides the number of countries in each region in a column called country_count
, the mean
happiness for each region in a column called mean_happiness
, and the mean
freedom of each region in a column called mean_freedom.
Store your resulting table in a variable called regional_stats_df.
# A tinble: 10 × 4
region country_count mean_happiness mean_freedom
<chr> <int> <dbl> <dbl>
1 Australia and New Zealand 2 7.28 0.645
2 Central and Eastern Europe 29 5.33 0.358
3 Eastern Asia 6 5.63 0.462
4 Latin America and Caribbean 22 6.14 0.502
5 Middle East and Northern Africa 20 5.41 0.362
6 North America 2 7.27 0.590
7 Southeastern Asia 9 5.32 0.557
8 Southern Asia 7 4.58 0.373
9 Sub-Saharan Africa 40 4.20 0.366
10 Western Europe 21 6.69 0.550
13.
[Challenge Problem] Compare the average gdp per capita of the ten least
happy Western European countries with the ten happiest
Sub-Saharan African countries. For testing, you can store the resulting data.frame or table as gdp_df
. # A tibble: 1 × 2
europe_gdp africa_gdp
Your preview ends here
Eager to read complete document? Join bartleby learn and gain access to the full version
- Access to all documents
- Unlimited textbook solutions
- 24/7 expert homework help
Related Questions
The exercise involving data in this and subsequent sections were designed to be solved using Excel. Johnson Filtration, Inc. provides maintenance service for water-filtration systems. Suppose that in addition to information on the number of months since the machine was serviced and whether a mechanical or an electrical repair was necessary, the managers obtained a list showing which repairperson performed the service. The revised data follow.
Repair Time(hours)
Months SinceLast Service
Type ofRepair
Repairperson
2.9
2
electrical
Dave Newton
3.0
6
mechanical
Dave Newton
4.8
8
electrical
Bob Jones
1.8
3
mechanical
Dave Newton
2.9
2
electrical
Dave Newton
4.9
7
electrical
Bob Jones
4.2
9
mechanical
Bob Jones
4.8
8
mechanical
Bob Jones
4.4
4
electrical
Bob Jones
4.5
6
electrical
Dave Newton
Ignore for now the months since the last maintenance service (x1 ) and the repairperson…
arrow_forward
Identify the feature of the data that would be missed if part (b) was completed without constructing the scatterplot. Choose the correct answer below
arrow_forward
Some analysts complain that spreadsheet models are difficult to resize. You can be the judge of this. Suppose the current product mix problem is changed so that there is an extra resource, packaging labor hours, and two additional PC models, 9 and 10. What additional input data are required? What modifications are necessary in the spreadsheet model (including range name changes)? Make up values for any extra required input data and incorporate these into a modified spreadsheet model. Then optimize with Solver. Do you conclude that it is easy to resize a spreadsheet model? (By the way, it turns out that algebraic models are typically much easier to resize.)
arrow_forward
Write a report of the different research design with exceptional of experimental research design.
arrow_forward
The Excel file for this assignment contains a database with information about the tax assessment value assigned to medical office buildings in a city. The following is a list of the variables in the database:
FloorArea: square feet of floor space
Offices: number of offices in the building
Entrances: number of customer entrances
Age: age of the building (years)
AssessedValue: tax assessment value (thousands of dollars)
Use the data to construct a model that predicts the tax assessment value assigned to medical office buildings with specific characteristics.
Construct a scatter plot in Excel with FloorArea as the independent variable and AssessmentValue as the dependent variable. Insert the bivariate linear regression equation and r^2 in your graph. Do you observe a linear relationship between the 2 variables?
Use Excel’s Analysis ToolPak to conduct a regression analysis of FloorArea and AssessmentValue. Is FloorArea a significant predictor of AssessmentValue?
Construct a scatter plot…
arrow_forward
The table gives the first 5 observations of 42 years of data on boats registered in Florida and manatees killed by boats.
Year
Boats
Manatees
1977
447
Florida manatees killed by boats
140-
120-
100-
80-
1978
40
1979
20
1980
1981
1982
460
481
498
513
512
To access the data, click the link for your preferred software format.
CSV Excel (xls) Excel (xlsx) JMP Mac-Text Minitab14-18 Minitab18+ PC-Text R SPSS TI CrunchIt!
The scatterplot of this data shows a strong positive linear relationship.
13
21
This scatterplot has a linear
(straight-line) overall pattern.
24
16
24
20
Moore/Nott, The Basic Practice of Statistics, 9⁹, 2021 W. H. Freeman and Company
nh
arrow_forward
Now monitor the process. An additional ten days of data have been collected, see table labeled “1st 10 Days of Monitoring Reservation Processing Time” in the Data File.
Develop Xbar and R charts for the 1st 10 days of monitoring. Plot the data for the 1st 10 days on the Xbar and R charts.
Is the process in control? If the control chart indicates an out-of-control process, note which days, the pattern, and whether it is the Xbar or R chart.
Based on the X-bar and R Charts that you developed for the 1st 10 days of data, is the process in control?
Group of answer choices
No. The X-bar and R Charts are both out of control.
No. The X-bar Chart is in control, but the R Chart is out of control.
No. The R Chart is in control, but the X-bar Chart is out of control.
Yes. The X-bar and R Charts are both in control.
arrow_forward
A foldable
arrow_forward
Using the given result/data, create a discussion with backup related literature.
arrow_forward
The r code for side by side boxplot of vitamind v newage and vitamin d v country.
Scatterplot code for relationship between vitamin d level and age.
arrow_forward
The migration pattern of Monarch butterflies are tracked by a catch-and-release method in which individual
butterflies are tagged with a circular, lightweight sticker placed carefully on the wings so as not to impede
their ability to fly. The sticker contains a unique ID number. Volunteers across the U.S. and South America
capture the butterflies, record the IDs if they are tagged, and release them. This allows us to track the
locations each unique ID is found, allowing us to estimate the migration pattern. On average, 1 out of 100
captured butterflies are already tagged. Suppose you are a volunteer and capture 50 butterflies; let X denote
the number of those that are already tagged. What is the distribution of X? What is the probability that
you catch at least one tagged butterfly?
arrow_forward
Please recheck and provide clear and complete step-by-step solution in scanned legible handwriting or computerized output
arrow_forward
SEE MORE QUESTIONS
Recommended textbooks for you
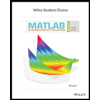
MATLAB: An Introduction with Applications
Statistics
ISBN:9781119256830
Author:Amos Gilat
Publisher:John Wiley & Sons Inc
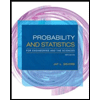
Probability and Statistics for Engineering and th...
Statistics
ISBN:9781305251809
Author:Jay L. Devore
Publisher:Cengage Learning
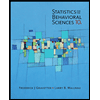
Statistics for The Behavioral Sciences (MindTap C...
Statistics
ISBN:9781305504912
Author:Frederick J Gravetter, Larry B. Wallnau
Publisher:Cengage Learning
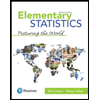
Elementary Statistics: Picturing the World (7th E...
Statistics
ISBN:9780134683416
Author:Ron Larson, Betsy Farber
Publisher:PEARSON
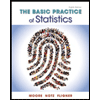
The Basic Practice of Statistics
Statistics
ISBN:9781319042578
Author:David S. Moore, William I. Notz, Michael A. Fligner
Publisher:W. H. Freeman
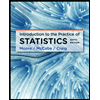
Introduction to the Practice of Statistics
Statistics
ISBN:9781319013387
Author:David S. Moore, George P. McCabe, Bruce A. Craig
Publisher:W. H. Freeman
Related Questions
- The exercise involving data in this and subsequent sections were designed to be solved using Excel. Johnson Filtration, Inc. provides maintenance service for water-filtration systems. Suppose that in addition to information on the number of months since the machine was serviced and whether a mechanical or an electrical repair was necessary, the managers obtained a list showing which repairperson performed the service. The revised data follow. Repair Time(hours) Months SinceLast Service Type ofRepair Repairperson 2.9 2 electrical Dave Newton 3.0 6 mechanical Dave Newton 4.8 8 electrical Bob Jones 1.8 3 mechanical Dave Newton 2.9 2 electrical Dave Newton 4.9 7 electrical Bob Jones 4.2 9 mechanical Bob Jones 4.8 8 mechanical Bob Jones 4.4 4 electrical Bob Jones 4.5 6 electrical Dave Newton Ignore for now the months since the last maintenance service (x1 ) and the repairperson…arrow_forwardIdentify the feature of the data that would be missed if part (b) was completed without constructing the scatterplot. Choose the correct answer belowarrow_forwardSome analysts complain that spreadsheet models are difficult to resize. You can be the judge of this. Suppose the current product mix problem is changed so that there is an extra resource, packaging labor hours, and two additional PC models, 9 and 10. What additional input data are required? What modifications are necessary in the spreadsheet model (including range name changes)? Make up values for any extra required input data and incorporate these into a modified spreadsheet model. Then optimize with Solver. Do you conclude that it is easy to resize a spreadsheet model? (By the way, it turns out that algebraic models are typically much easier to resize.)arrow_forward
- Write a report of the different research design with exceptional of experimental research design.arrow_forwardThe Excel file for this assignment contains a database with information about the tax assessment value assigned to medical office buildings in a city. The following is a list of the variables in the database: FloorArea: square feet of floor space Offices: number of offices in the building Entrances: number of customer entrances Age: age of the building (years) AssessedValue: tax assessment value (thousands of dollars) Use the data to construct a model that predicts the tax assessment value assigned to medical office buildings with specific characteristics. Construct a scatter plot in Excel with FloorArea as the independent variable and AssessmentValue as the dependent variable. Insert the bivariate linear regression equation and r^2 in your graph. Do you observe a linear relationship between the 2 variables? Use Excel’s Analysis ToolPak to conduct a regression analysis of FloorArea and AssessmentValue. Is FloorArea a significant predictor of AssessmentValue? Construct a scatter plot…arrow_forwardThe table gives the first 5 observations of 42 years of data on boats registered in Florida and manatees killed by boats. Year Boats Manatees 1977 447 Florida manatees killed by boats 140- 120- 100- 80- 1978 40 1979 20 1980 1981 1982 460 481 498 513 512 To access the data, click the link for your preferred software format. CSV Excel (xls) Excel (xlsx) JMP Mac-Text Minitab14-18 Minitab18+ PC-Text R SPSS TI CrunchIt! The scatterplot of this data shows a strong positive linear relationship. 13 21 This scatterplot has a linear (straight-line) overall pattern. 24 16 24 20 Moore/Nott, The Basic Practice of Statistics, 9⁹, 2021 W. H. Freeman and Company nharrow_forward
- Now monitor the process. An additional ten days of data have been collected, see table labeled “1st 10 Days of Monitoring Reservation Processing Time” in the Data File. Develop Xbar and R charts for the 1st 10 days of monitoring. Plot the data for the 1st 10 days on the Xbar and R charts. Is the process in control? If the control chart indicates an out-of-control process, note which days, the pattern, and whether it is the Xbar or R chart. Based on the X-bar and R Charts that you developed for the 1st 10 days of data, is the process in control? Group of answer choices No. The X-bar and R Charts are both out of control. No. The X-bar Chart is in control, but the R Chart is out of control. No. The R Chart is in control, but the X-bar Chart is out of control. Yes. The X-bar and R Charts are both in control.arrow_forwardA foldablearrow_forwardUsing the given result/data, create a discussion with backup related literature.arrow_forward
- The r code for side by side boxplot of vitamind v newage and vitamin d v country. Scatterplot code for relationship between vitamin d level and age.arrow_forwardThe migration pattern of Monarch butterflies are tracked by a catch-and-release method in which individual butterflies are tagged with a circular, lightweight sticker placed carefully on the wings so as not to impede their ability to fly. The sticker contains a unique ID number. Volunteers across the U.S. and South America capture the butterflies, record the IDs if they are tagged, and release them. This allows us to track the locations each unique ID is found, allowing us to estimate the migration pattern. On average, 1 out of 100 captured butterflies are already tagged. Suppose you are a volunteer and capture 50 butterflies; let X denote the number of those that are already tagged. What is the distribution of X? What is the probability that you catch at least one tagged butterfly?arrow_forwardPlease recheck and provide clear and complete step-by-step solution in scanned legible handwriting or computerized outputarrow_forward
arrow_back_ios
arrow_forward_ios
Recommended textbooks for you
- MATLAB: An Introduction with ApplicationsStatisticsISBN:9781119256830Author:Amos GilatPublisher:John Wiley & Sons IncProbability and Statistics for Engineering and th...StatisticsISBN:9781305251809Author:Jay L. DevorePublisher:Cengage LearningStatistics for The Behavioral Sciences (MindTap C...StatisticsISBN:9781305504912Author:Frederick J Gravetter, Larry B. WallnauPublisher:Cengage Learning
- Elementary Statistics: Picturing the World (7th E...StatisticsISBN:9780134683416Author:Ron Larson, Betsy FarberPublisher:PEARSONThe Basic Practice of StatisticsStatisticsISBN:9781319042578Author:David S. Moore, William I. Notz, Michael A. FlignerPublisher:W. H. FreemanIntroduction to the Practice of StatisticsStatisticsISBN:9781319013387Author:David S. Moore, George P. McCabe, Bruce A. CraigPublisher:W. H. Freeman
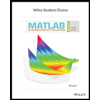
MATLAB: An Introduction with Applications
Statistics
ISBN:9781119256830
Author:Amos Gilat
Publisher:John Wiley & Sons Inc
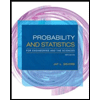
Probability and Statistics for Engineering and th...
Statistics
ISBN:9781305251809
Author:Jay L. Devore
Publisher:Cengage Learning
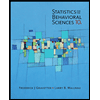
Statistics for The Behavioral Sciences (MindTap C...
Statistics
ISBN:9781305504912
Author:Frederick J Gravetter, Larry B. Wallnau
Publisher:Cengage Learning
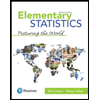
Elementary Statistics: Picturing the World (7th E...
Statistics
ISBN:9780134683416
Author:Ron Larson, Betsy Farber
Publisher:PEARSON
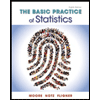
The Basic Practice of Statistics
Statistics
ISBN:9781319042578
Author:David S. Moore, William I. Notz, Michael A. Fligner
Publisher:W. H. Freeman
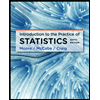
Introduction to the Practice of Statistics
Statistics
ISBN:9781319013387
Author:David S. Moore, George P. McCabe, Bruce A. Craig
Publisher:W. H. Freeman